I’ve been in business for several years now, and I’m beginning to amass some personal history with respect to how long certain tasks take. If I have 5-6 times on say, how long it takes to install baseboard per lin. ft., how do I handle all the info in the database. How do the rest of you handle it? Do you just average out the results?
Discussion Forum
Discussion Forum
Up Next
Video Shorts
Featured Story
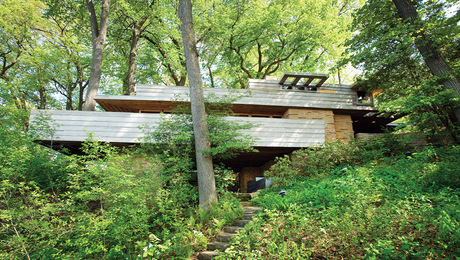
Wright's Pew House on Lake Mendota in Wisconsin recalls the architect’s famed Fallingwater, but on a more modest scale. It found its steward.
Highlights
"I have learned so much thanks to the searchable articles on the FHB website. I can confidently say that I expect to be a life-long subscriber." - M.K.
Replies
Generally, the mean is a more indicative number than the average and one common practise is to price to the mean of the data points above the mean (i.e. plot the mean, discard the points lower than the mean, then plot the mean again, there are variations, such as discarding 25% of your lowest data points). But the key word there was "plot"; and you'd want to plot against several other variables looking for correlationships (I've made a giant assumption here that you have neither the software, nor the inclination, to data-mine for correlationships as you gain further data points; but, you can wheel around basic 123 or Exel). For a small company and few results, sometimes you can just plot your points against physical jobs and SWAG the answers; however, plotting results against a few factors (e.g. crew size, job size, time of year, materials, new vs old construction, length of job, some sort of crew skill variable, etc.) can often yield surprising results when you line them all up. Don't forget commonly over-looked factors (e.g. number of job arrivals/setups, hours worked/day, haul distance, etc.).
If you're good at interpreting visual data, consider using 3-axis plots and decide if you want to investigate anomalies (like when you have most jobs clustered and one or two out of range) or the norm (this would apply most when you get scattered results with no clear cluster).
Phill Giles
The Unionville Woodwright
Unionville, Ontario
I've recently purchased some Add-In software for Excel. See http://www.add-ins.com/ For their Analysis Suite.
I think I have the software in place and a fair understanding of Excel. Where do we go from here? I mean, what would be a more thorough analysis?
I'm in the process of applying what Harold Kerzner, in his tome Project Management, calls a Lessons Learned File to the numbers in the database. This may help in qualifying some of the data.
Obviously I'm not too concerned with data with a tight point spread, like Bob describes. What concerns me is the areas where there are wide differences - for me, framing. Our framing projects tend to vary considerably in size and shape, and while the crew members, weather, and site logistics may come into play, there's probably more attributable to the inherent design. One-piece baseboard, on the other hand, is more the same from house to house regardless of the profile.
If I calculate the average (mean), I'm concerned about the spread between it and the outlying data points. I'm not sure recalculating the mean or finding the median addresses this much better. The margin of error is still great enough to impact the estimated price and schedule. If I do go with this method, however, do I add a percentage as a fudge factor for the possibility that the task might still come in at the upper end of my database? If so what method do I use to calculate that percentage.
>The margin of error is still great enough to impact the estimated price and schedule.
No matter what metric you use, you'll have a problem if you don't have a thorough understanding of what is causing the differences in the underlying data. Using myself as an example, there's a commodity that I was told by the supplier to estimate at $2/sf. On one job it was 1.97 and on the next it was 3.25. Unless the gap can be understood and explained, that data will be useless (or nearly so) in a numerical analysis.
If you want to get even more technical about it, you can apply confidence intervals to your data....i.e., the cost should be x ± y with z% confidence. But 5 or 6 data points will not provide enough info to do that well. Would work on thousands of data points, where the outliers had limited impact, but not here. I think the best you can do is capture the data, calculate some metrics over time to see if you can spot trends, but for new jobs try to identify a similarity to a prior job, and bias toward that number.
An observer would say that statisticians are just math-monkeys; however, the real art is in figuring out what to analyze. You also have to make some decisions as to what your goals are.
CAVEAT, my background is large corporation/high-volumes
a) There are basically 2 outcome sets that you are shooting for: set 1 is to make a profit every time out of the box (within statistical confidence; this confidence would be something like "9 times out of 10, every year", or "9 times out of 10, rolling average over a 3 year span", ...); set 2 is to make a profit within a set period (per year, per rolling year, multi-years, ...) without regard to the number of successful single incidents. The interesting thing here is that the more confidence you have in set 2, the more risks you can take with set 1.
b) And there are 3 factor groups at work here: resource costs (units of labour, materials, overheads), environmental influences (job size, complexity, design, skills match, weather, ...), and margin. And then there's "TIME"! The only lever you can just reach out and pull is margin, so you're looking to control resources and measure the environment so that you can predict time. You'll also need to set the granularity of your tracked activities, do you want to measure "trim" or divide that into "doors", "windows", & "base"
c) IMHO and per my 1st post, the first step is to run some simple plots (aka: charts) on the data to see what it looks like. Working in a large corporation, as I did, you soon learn that everything eventually has to be explained to a salesman once you get it past the accountants: what that means is, "simple is better". I like to start with plotting my major data points against a common base. In your case, I'd start with "physical job" as the X-axis for one series of charts and "unit cost" for a second series.
_________________________________________________________
Once you've done all this, you get to decide which variables need the most attention at the prediction stage and what to track during exection. As everyone has said (I think), you'll be wanting to look at the greatest deviation first: fix (or accurately predict) the big swings and then go looking for the small stuff. That's what charting is supposed to do: convert all those numbers into pictures so you can find what you're looking for.
This post is already too long. I'm not familiar with your add-on; I liked the descriptions for the chart-helper type of add-ons, I'd have to use macros for creating complex charts, etc. before commenting..
Phill Giles
The Unionville Woodwright
Unionville, Ontario
Certainly I intend to make a profit, This goes without saying. If that was the only goal, I could throw out all data and just retain the highest values, building the estimate on those.
However, I must also be competetive, and not only with other builders that know there costs, but the ones that aren't charging nearly enough.
The detail needed to track the cause that is driving the variance seems like a difficult task to master. While there may look like some obvious culprits at first blush, the chances are that it's a composite of things driving a chain of events:
Weather
New crew member
Talkative customer, subs, or employees (or all three)
Personal problems outside of work
Site logistics
Unavailable materials or equipment
Sick or not feeling well
Spring fever
Complex design
Unforeseen work items
Excessive material handling
The list could go on and on.
My point is that the cause of the variance may be a mixture of the events above and more. Do you track it saying it was 45% weather related, 5% lack of equipment, 28% talkative customer, and 22% belly ache? If so, the tracking would not be worth the effort, since there are so many variables working against us and a multitude of combinations.
Why not accept that performance will hover between two extremes with the majority of data points at some given point? Base the task estimate not only on this single point, but also on the spread of the outliers. The wider the spread, the more risk inherent in the task. In other words, build a contingency value ($) based upon the risk associated with each and every task, rather than some arbitrary percentage at the end of the estimating process based upon some gut feeling of performance.
You could build a multiple regression based on all of those factors and more. But the question is, while it would conceivably make you more precise, would it make you more accurate?
Pete, you don't have enough data points to even consider tracking that many variables. IMHO, you have to look for the anomalies, and then decide what the key factors were to drive the aberation. As I suggested in my first post, if your data points are fairly well clustered, you can just plot a mean that's adjusted for your risk envelope (most guys I know can pretty much eyeball that from their first chart).
Look back at my comments on the two outcome sets. Outcome 2 is all about long-term profit and allows you to cut closer to the bone for outcome 1 to be competitive.
But please, do some simple plots, probably unit cost/job, ask yourself what 3 factors caused the highest cost, and then plot them to see if there's a high degree of correlation and therefore can be applied meaningfully to the rest of the jobs. There are no silver bullets and you can't compete with someone who is losing money..
Phill Giles
The Unionville Woodwright
Unionville, Ontario
Pete-
I'd say it depends on the variations within the data. If, for example, you had 6 numbers for hanging a door that ranged from 1 hour to 1.1 hours, you're find with using 1.05, or even 1.0, for your figure. If you have six numbers that range from 1 to 2.5 hours, you'd better have a look at what drove the number in each case- the 2.5 hour number may have been due to tight quarters on that job, or out of plumb walls, or varying wall thicknesses- that makes it the "exception" rather than the "norm".
While Phil offers very valid methods regarding finding the mean, and then discarding the mean and redistributing, etc., I think that's a wee bit more than the average contractor needs to do- the goal is to get data that covers your "typical" application, and then apply adjustments based on site conditions.
Bob
If I had a bunch of items, each with only a half dozen data points or so, I would probably just stick them in an Excel spreadsheet and eyeball the relationship between the numbers. Means and even medians can be rather misleading if you data is highly skewed. If you have four job where it took an hour each time and one in which it took six hours, then the mean would say 2 hours, which was double the time of four out of the five jobs.
Median measures can also be misleading if the data are heavily skewed, if you had values of 1, 2, 3, 10, 12 then the median would give you 3 while the mean would be 7. Neither of which really tells you much about what was really going on. I agree with the previous comment about understanding why the values vary, if they do. If they don't you don't need to do much analysis. If they do vary widely, just doing means and/or medians probably isn't going to add much understanding. Unless you are doing enough volume to just let things wash out in the long run, I think the analysis needs to focus on the factors that make up the differences.
If you start accumulating more data, then loading it into Access can give you more analytical ability, but you need to learn something about using access (I assume that most business people already know something about Excel.)
Both Excel and Access have "Wizards" to help create what Microsoft calls "Charts" (graphs to most of us). When working with a limited number of data points, however, Excel is much easier for most people to use.